Genome Wide Association Studies
Genome wide association studies (GWAS) are studies of the genome with the aim to interrogate common genetic variants in association with disease (specific phenotype). GWAS investigate the entire genome, comparing a large group of individuals with a phenotype of interest to individuals without the phenotype. The associations studies are Single-Nucleotide Polymorphisms (SNPs).
The aim of GWAS are to
- Identify genetic variants to explain differences in phenotype among individuals in a poluation
- Qualitative - disease status, presence / absence of a congenital defect
- Quantitative - blood glucose level, % body fat
- If association is present, follow up study is required. GWAS do not express causative relationships.
- Understand etiology of disease
- Characterize relevance in general population
- Deliver informative prevention and treatment strategies
General Process of GWAS
- Large cohort of cases and controls (n > 1000)
- Matched for confounding variables such as race ethnicity and sex
- Microarray-based SNP genotyping
- ~1 million random marker SNPs or ~25,000 risk-enhancing SNPs (e.g. nsSNP)
- Derivation of haplotypes
- Predicted on International HapMap
- Detection of association signals
- χ2 or similar test
- Replication of association
- Biological validation of association
Strategy in GWAS: sample type
Match case-control samples (age, sex, demographics)
Sample size - The larger the better
GWAS Assumptions
- Bi-allelic SNPs
- Locus in a genome that contains two observed alleles, counting the reference as one, thus allowing for one variant allele. This is compared to multi-allelic sites, locuses that contain three or more observed alleles (such as blood group in humans), counting the reference as one, thus allowing for two or more variant alleles.
- Common Ancestors
- Linkage disequilibrium and haplotypes
- Common disease-common variant
Software used for GWAS analysis
- Plink - developed by MIT only available for Linux
- SNPassoc - developed by Juan R. Gonzalez, published in Bioinformatics, Volume 23, Issue 5
- GenABEL - developed by Aulchenko et al., published in Bioinformatics, Volume 23, Issue 10
Statistical Tests
- Case-control
- Allelic Chi square test
- Cochran-Armitage trend test
- Logistic regression
- Quantitative traits
- Linear regression
- Co-variate iterations
- Age, sex, etc
Related Literature
- Norrgard, K. (2008) Genetic variation and disease: GWAS. Nature Education 1(1):87
- Norrgard , K. & Schultz, J. (2008) Using SNP data to examine human phenotypic differences. Nature Education 1(1):85
First discoveries of GWAS
- 2005 - Learned through GWAS that age-related macular degeneration is associated with variation in the gene for complement factor H, which produces a protein that regulates inflammation [Klein et al. (2005) Science, 308, 385-389]
- 2007 - the Wellcome Trust Case-Control Consortium (WTCCC) carried out GWAS for the diseases coronary heart disease, type I diabetes, type 2 diabetes, rheumatoid arthritis, Crohn's disease, bipolar disorder and hypertension.
Examples of GWAS
- Association scan of 14,500 nonsynonymous SNPs in four diseases identifies autoimmunity variants. [Nat Genet. 2007]
- Genome-wide association study of 14,000 cases of seven common diseases and 3,000 shared controls. Wellcome Trust Case Control Consortium [Nature. 2007;447;661-78]
- Genomewide association analysis of coronary artery disease. Samani et al. [N Engl J Med. 2007;357;443-53]
- Sequence variants in the autophagy gene IRGM and multiple other replicating loci contribute to Crohn's disease susceptibility. Parkes et al. [Nat Genet. 2007;39;830-2]
- Robust associations of four new chromosome regions from genome-wide analyses of type 1 diabetes. Todd et al. [Nat Genet. 2007;39;857-64]
- A common variant in the FTO gene is associated with body mass index and predisposes to childhood and adult obesity. Frayling et al. [Science. 2007;316;889-94]
- Replication of genome-wide association signals in UK samples reveals risk loci for type 2 diabetes. Zeggini et al. [Science. 2007;316;1336-41]
- A genome-wide association study of type 2 diabetes in Finns detects multiple susceptibility variants. Scott et al. [Science, 316, 1341–1345.]
International HapMap Project
- Let's talk about Linkage Disequilibrium (LD) across the genome in several populations
- Genotyped ~4 million SNPs on samples of African, eastAsian, European ancestry
Some terminology
- SNP - DNA sequence variations that occur when a single nucleotide is altered
- Genotypes - pair of alleles (one paternal, one maternal)
- Haplogroup - a group of closely-linked SNPs
- Haplotype - specific sequence of alleles along a single chromosome: AT and CT
Most commonly studied haplogroups in human
- Y-DNA haplogroups; passed solely along patrilineal line
- Haplogroups defined by non-recombinant DNA on Y
- mt-DNA haplogroups; passed solely along matrilineal line
Linkage Disequilibrium
- LD is the non-random association between alleles at different loci
- Created by evolutionary force
- Close genetic loci exhibit strong LD
- The larger the population size, the weaker LD for a given genetic distance
Confounding interpretations of GWAS; Population Stratification
- At identified risk loci, there are multiple alleles associated with disease at a wide frequency
- Presence of pleiotropy; same variant can be associated with multiple traits
- For an associated SNP, GWAS can not distinguish if:
- Association signal is caused by a rare variant of large effect
- Association signal is caused by common variant with small effect
GWAS Summary
- Linkage analysis using families takes unbiased look at whole genome, but fails to identify reliably complex trait loci in pedigree analysis
- Due to small size of genetic effectsfor many complex genetic traits
- Candidate gene association studies have greater power to identify smaller genetic effects, but need prior knowledge about etiology of disease
- Genome-wide association studies combine the genomic coverage of linkage analysis with the power of association to have much better chance of finding complex trait susceptibility variants
- GWAS is unbiased with respect to prior knowledge about etiology of disease
- GWAS is unbiased with respect to genome location
- Statistical analysis and interpretation of data are imperative
What comes next?
Post-GWAS functional studies are lagging
In 2016 - there have been 3835 GWAS studies, versus 84 functional studies.
Confounding factors in establishing function post GWAS "Linking association to causation"
- ‘‘one-gene, one-mutation, one-outcome’’ model does not always apply
- Many diseases are not caused by single mutations (e.g. cardiovascular disease, cancer, Alzheimer’s disease, Parkinson’s disease, and type 2 diabetes)
- Genes may act in epistatic fashion
- Genes may act in additive fashion
- Genes function may be influenced by complex regulatory mechanisms
- Linking associated variant in non-coding regions to target genes is complicated
CRISPR-Case9
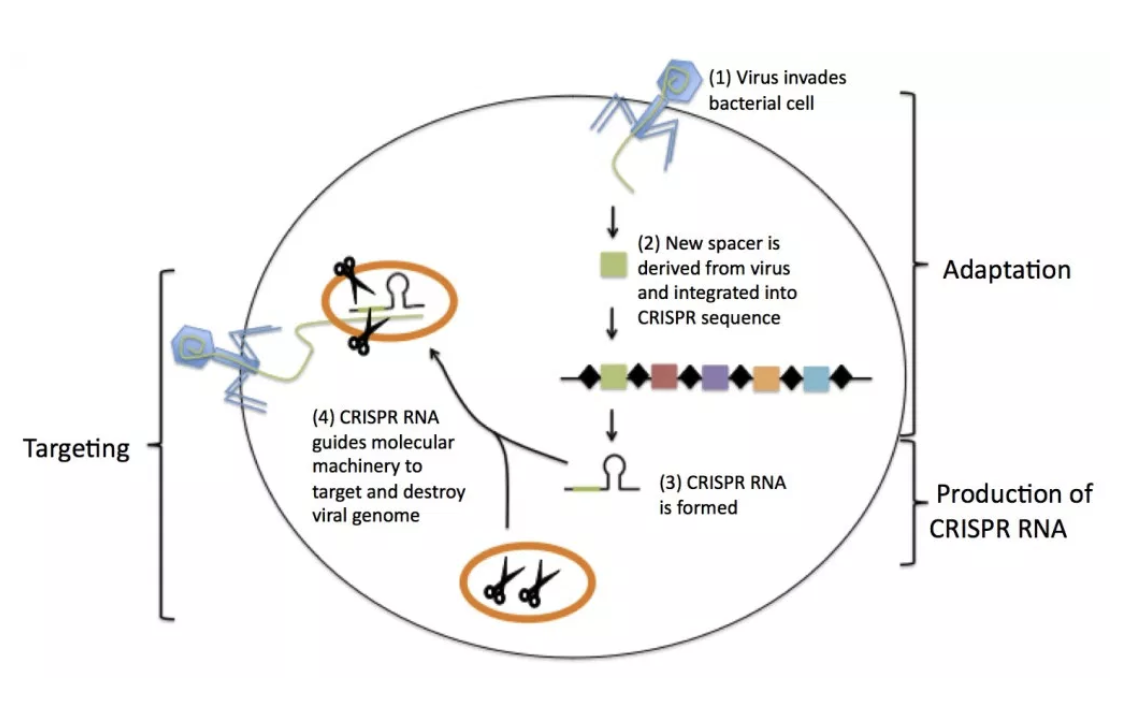
Figure creidt - Pak, E. Harvard Medical School
Investigating candidate causal variants with CRISPR technology
- GWAS identified BCL11A associated with fetal hemoglobin (HbF)
- CRISPR used to “edit” a 10kb intronicregion in the mouse ortholog of BCL11A
- Dramatic reduction of Bc11 expression and increase of embryonic b-globin
- The region is a functional Bcl11a enhancer required for repression of embryonic b-globin
Determining Regulatory Variants' Target Genes
- Organization of genome in the nucleus
- Genome loops (physical contact) important in transcriptional regulation and disease
- Studying long-range contacts in the genome [e.g chromosome conformation capture (3C) techniques]
- 3D organization of chromatin
- Example: Association of intronic genetic variants at the FTO locus with obesity
- Studies showed obesity-associated region interacts with a specific genes promoter (Irx3)
- CRISPR studies identifies additional target gene, RX5